Entidades
Ver todas las entidadesClasificaciones de la Taxonomía CSETv0
Detalles de la TaxonomíaProblem Nature
Specification
Physical System
Software only
Level of Autonomy
Low
Nature of End User
Amateur
Public Sector Deployment
No
Data Inputs
User votes
Clasificaciones de la Taxonomía CSETv1
Detalles de la TaxonomíaIncident Number
50
AI Tangible Harm Level Notes
It is not clear whether the Decentralized Autonomous Organization involves any AI systems.
Special Interest Intangible Harm
no
Date of Incident Year
2016
Date of Incident Month
06
Date of Incident Day
17
Informes del Incidente
Cronología de Informes
- Ver el informe original en su fuente
- Ver el informe en el Archivo de Internet
Reflexiones sobre el truco de DAO
Acabamos de vivir el escenario de pesadilla que nos preocupaba cuando pedimos una moratoria en The DAO: alguien explotó una debilidad en el código de The DAO para vaciar más de 2 millones ($ 40 millones de …

- Ver el informe original en su fuente
- Ver el informe en el Archivo de Internet
El valor de la moneda digital Ethereum se ha reducido drásticamente en medio de un aparente gran ataque dirigido a una organización con grandes tenencias de la moneda.
El precio por unidad cayó a $15 desde máximos históricos de $21,50 en ho…
- Ver el informe original en su fuente
- Ver el informe en el Archivo de Internet
Análisis del exploit DAO
phil daian
Así que estoy seguro de que todo el mundo ha oído hablar de las grandes noticias que rodean a la DAO y que un hacker se la llevó por una suma de 150 millones de dólares utilizando el exploit de envío recu…
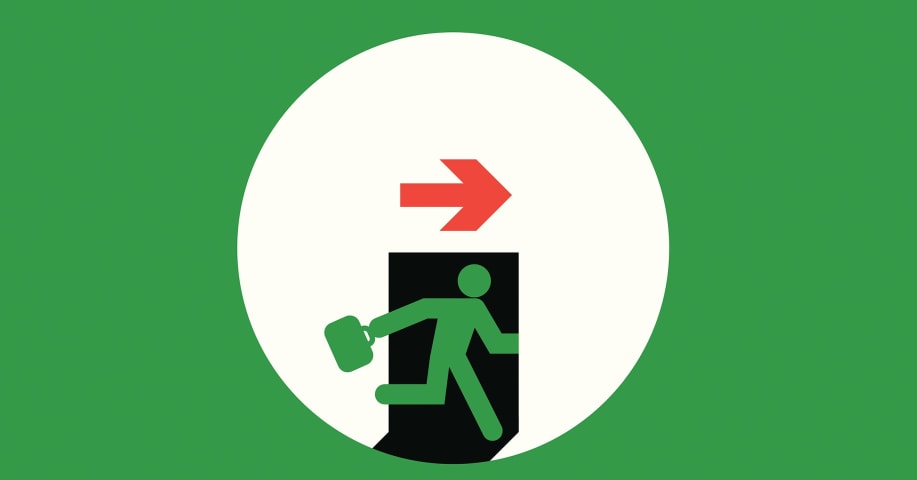
- Ver el informe original en su fuente
- Ver el informe en el Archivo de Internet
En algún momento de la madrugada del viernes, un ladrón se llevó 50 millones de dólares en moneda virtual.
Las víctimas son inversionistas en un fondo extraño llamado DAO, u Organización Autónoma Descentralizada, que invirtió más de $ 150 m…
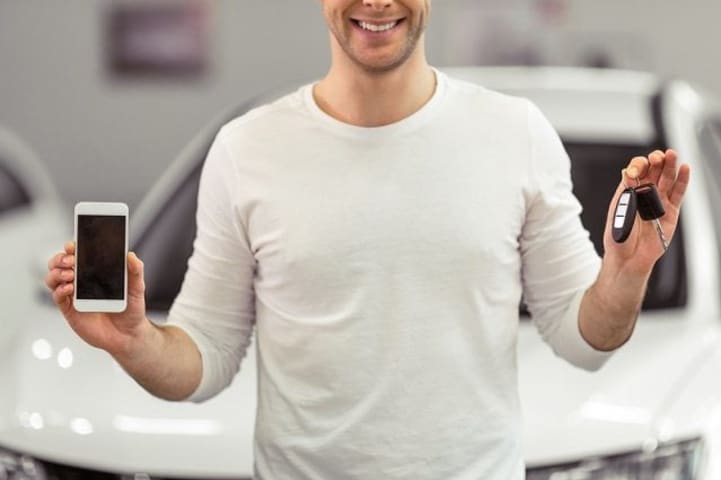
- Ver el informe original en su fuente
- Ver el informe en el Archivo de Internet
La reciente debacle que rodea a The DAO ha arrojado un foco interesante sobre la tecnología de contratos inteligentes. Dado que los desarrolladores individuales escribieron todo el concepto de este proyecto, parece que los contratos intelig…
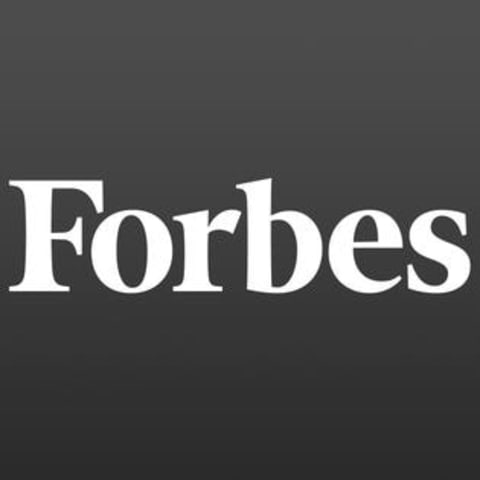
- Ver el informe original en su fuente
- Ver el informe en el Archivo de Internet
Ups. La primera Organización Autónoma Descentralizada de Ethereum, la DAO, ha perdido dinero, mucho. En concreto, ha perdido el equivalente a unos 60 millones de dólares. Esto no se debe a que sea una idea tonta y nadie se moleste con ella …
- Ver el informe original en su fuente
- Ver el informe en el Archivo de Internet
La trágica combinación de errores inevitables y código inmutable
La semana pasada fue testigo de un evento catastrófico en el ecosistema Ethereum, cuando The DAO, un contrato inteligente de menos de dos meses, comenzó a filtrar fondos rápid…
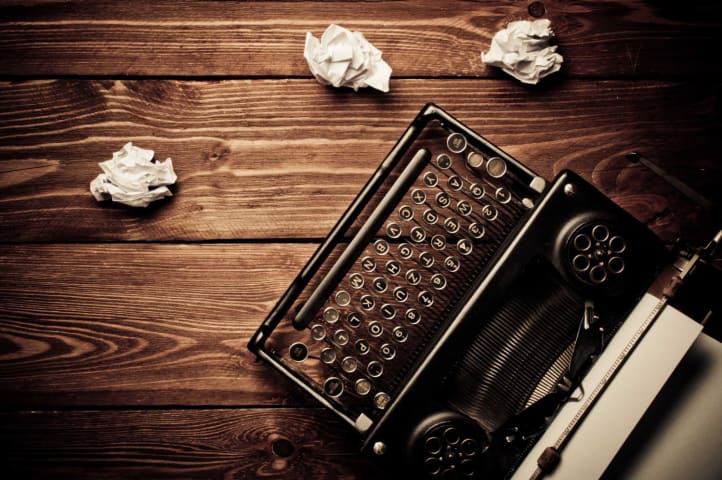
- Ver el informe original en su fuente
- Ver el informe en el Archivo de Internet
David Siegel es un orador y estratega de blockchain, fundador de Kryptodesign.com y curador de DecentralStation.com, un lugar para aprender sobre blockchain.
En este artículo, Siegal intenta ayudar a los periodistas a comprender qué sucedió…
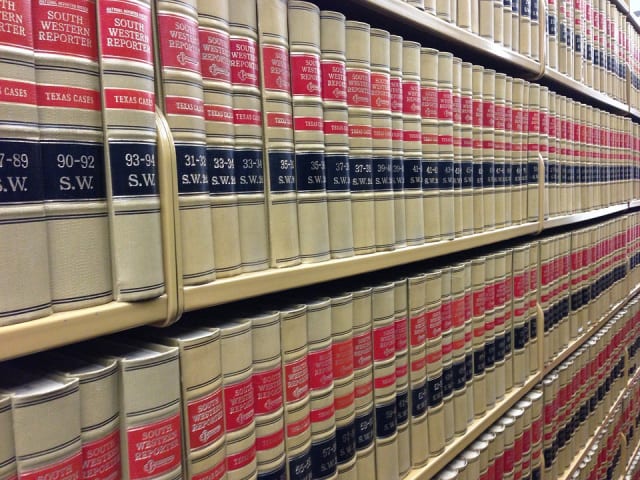
- Ver el informe original en su fuente
- Ver el informe en el Archivo de Internet
Blockchains, Smart Contracts y la Ley
…desentrañando los problemas legales que rodean a la DAO
Reuben Bramanathan Bloqueado Desbloquear Seguir Siguiendo 24 de junio de 2016
El debate público que siguió a la espectacular implosión de The DAO…
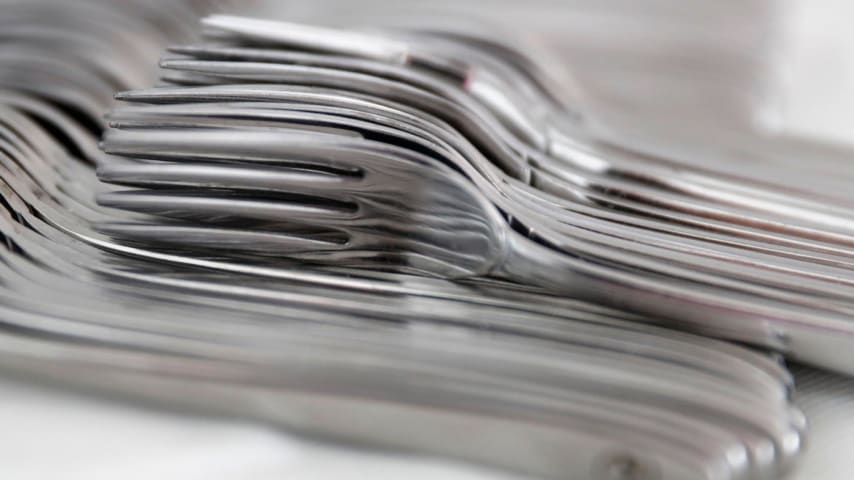
- Ver el informe original en su fuente
- Ver el informe en el Archivo de Internet
Ethereum irrumpió en la escena de las monedas virtuales hace casi un año. Es similar a bitcoin, pero con una diferencia clave. Además de admitir su propia moneda digital, ether, también admite contratos inteligentes, acuerdos escritos en có…
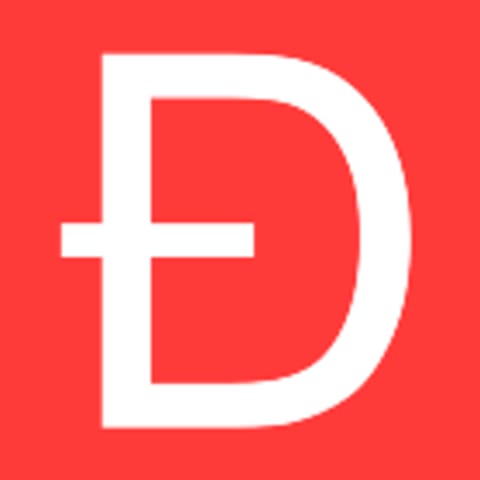
- Ver el informe original en su fuente
- Ver el informe en el Archivo de Internet
El Dao, el Hack, el Soft Fork y el Hard Fork Antonio Madeira 12 Mar 2019
¿Qué fue el incidente DAO?
En esta guía intentamos cubrir: cómo y por qué se creó The DAO, cómo se explotó The DAO, cómo la bifurcación suave falló miserablemente y po…
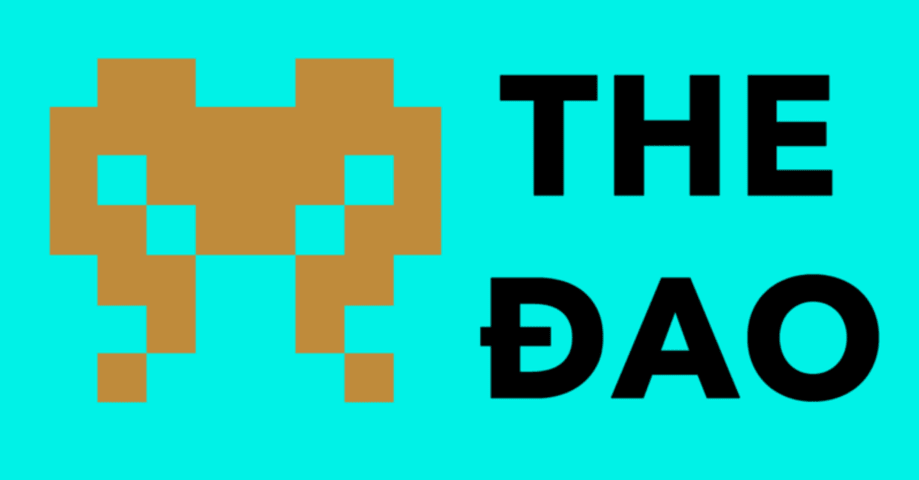
- Ver el informe original en su fuente
- Ver el informe en el Archivo de Internet
La historia de la DAO y las lecciones aprendidas
Christoph Jentzsch Bloqueado Desbloquear Seguir Siguiendo 24 de agosto de 2016
Hay algunas cosas que uno solo puede aprender a través de la experiencia, ya sea la propia o la de los demás. En…

- Ver el informe original en su fuente
- Ver el informe en el Archivo de Internet
El chico nuevo en el bloque
El Organismo Autónomo Descentralizado (DAO) estaba destinado a convertirse en el primer fondo de inversión descentralizado digital. Prácticamente todas sus operaciones diarias serían manejadas, bueno, virtualment…
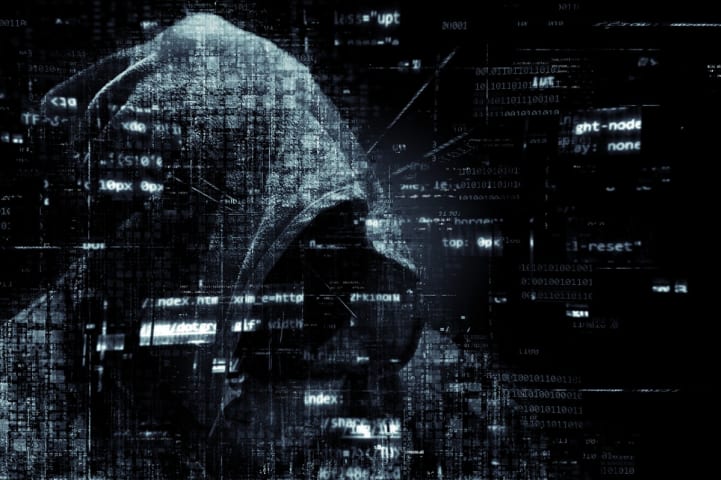
- Ver el informe original en su fuente
- Ver el informe en el Archivo de Internet
Ayer, un pirata informático realizó el segundo robo más grande en la historia de las monedas digitales.
Alrededor de las 12:00 PST, un atacante desconocido explotó una falla crítica en la billetera de múltiples firmas de Parity en la red Et…
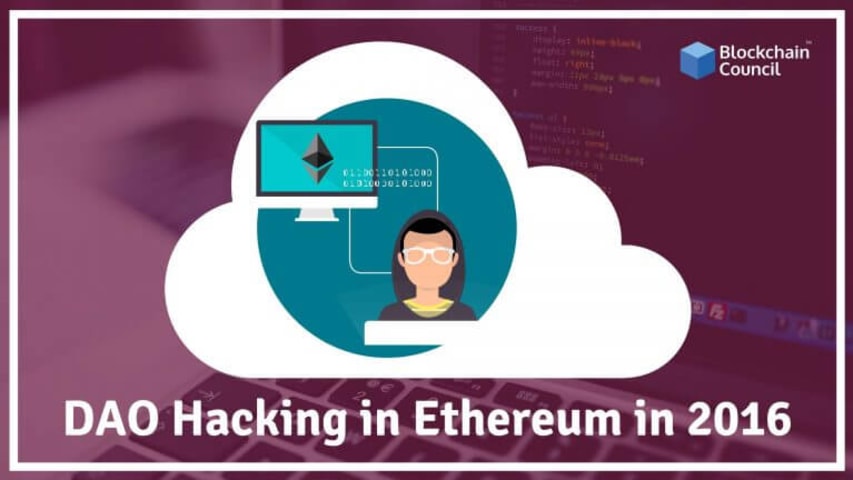
- Ver el informe original en su fuente
- Ver el informe en el Archivo de Internet
El hackeo de DAO que amenazó todo y afectó a Ethereum
¿Recuerdas cómo una organización autónoma descentralizada puede crear con el uso de Ethereum? En el año 2016, hubo una caída. Una startup estaba trabajando en un proyecto DOA llamado DAO…
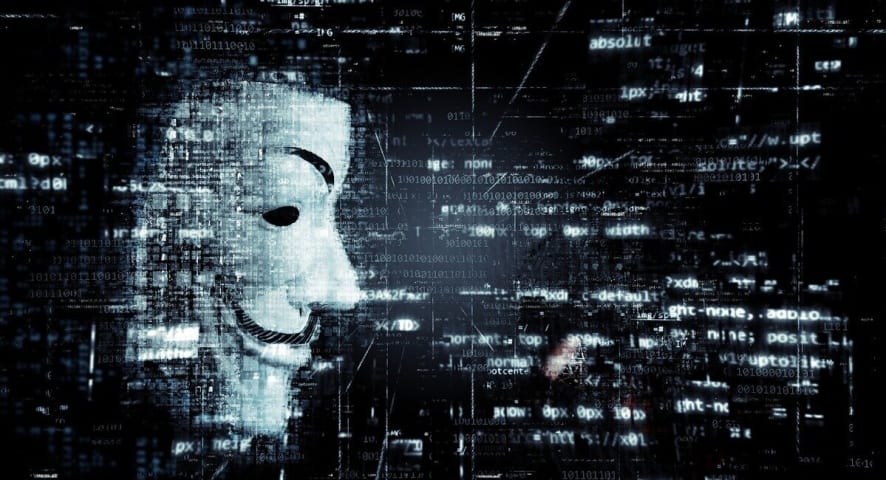
- Ver el informe original en su fuente
- Ver el informe en el Archivo de Internet
En 2016, una gran idea llegó a la red Ethereum. La Organización Autónoma Descentralizada (DAO) fue creada para operar como un fondo de capital de riesgo para proyectos descentralizados de criptomonedas. El DAO se creó como un contrato intel…
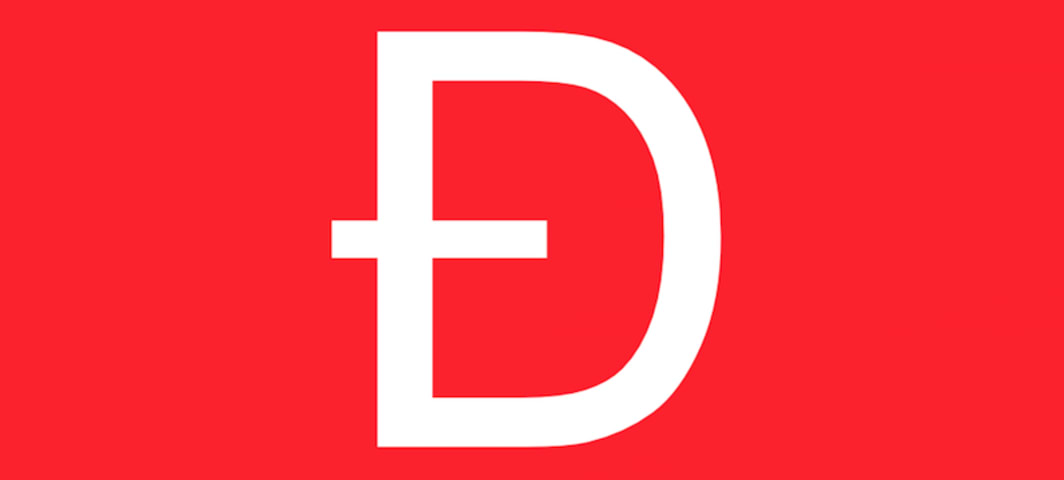
- Ver el informe original en su fuente
- Ver el informe en el Archivo de Internet
La Historia de la DAO — Su Historia y Consecuencias
Samuel Falkon Bloqueado Desbloquear Seguir Siguiendo 24 de diciembre de 2017
Uno de los conceptos más increíbles que se implementará con éxito a través de la tecnología blockchain es el DA…
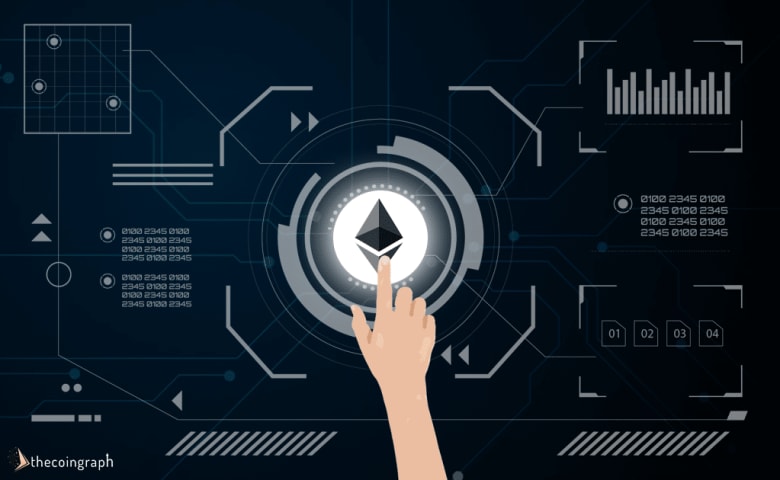
- Ver el informe original en su fuente
- Ver el informe en el Archivo de Internet
El truco de DAO: 50 millones de dólares robados y la bifurcación dura.
Cryptonomy Bloqueado Desbloquear Seguir Siguiendo 20 de abril de 2018
El último artículo explicaba la idea de las DAO, pero creo que no podemos hablar de las DAO sin men…
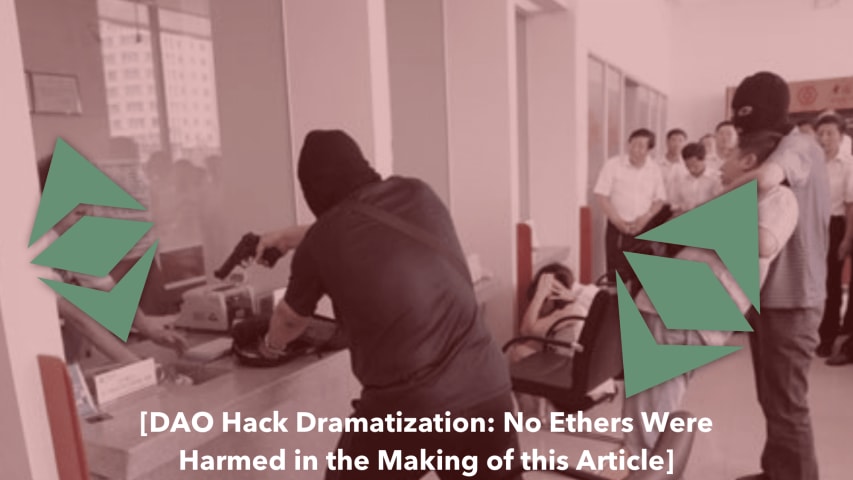
- Ver el informe original en su fuente
- Ver el informe en el Archivo de Internet
Las consideraciones de seguridad anulan todas las demás consideraciones en el software en general y específicamente en blockchain. Si la seguridad falla, nada más importa. Blockchain demuestra que las transacciones descentralizadas y sin co…
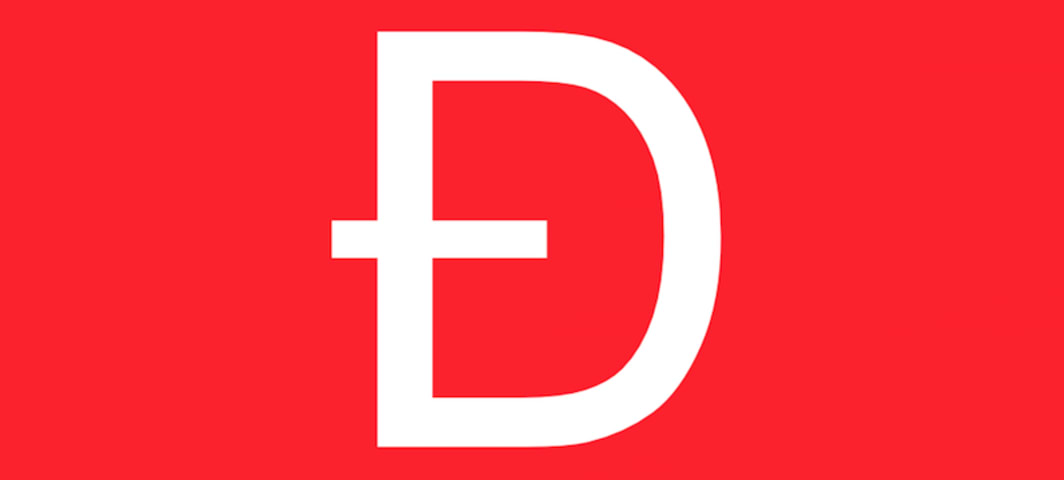
- Ver el informe original en su fuente
- Ver el informe en el Archivo de Internet
Explicación del hackeo de DAO: desafortunado despegue de los contratos inteligentes
Osman Gazi Güçlütürk Bloqueado Desbloquear Seguir Siguiendo 31 de julio de 2018
Logotipo de la DAO
Los contratos inteligentes trajeron organizaciones autóno…
- Ver el informe original en su fuente
- Ver el informe en el Archivo de Internet
CCN es un sitio de noticias financieras imparcial que informa sobre los mercados estadounidenses y las criptomonedas. Los artículos de opinión y las opiniones no deben atribuirse a CriptomonedaseICO. Los periodistas de CCN siguen un estrict…
- Ver el informe original en su fuente
- Ver el informe en el Archivo de Internet
Uno de los objetivos de diseño de Ethereum era simplificar la especificación de la capa de consenso. Ese es un objetivo noble, ya que facilita la reimplementación de la plataforma para diferentes lenguajes de programación y limitaciones. Pe…
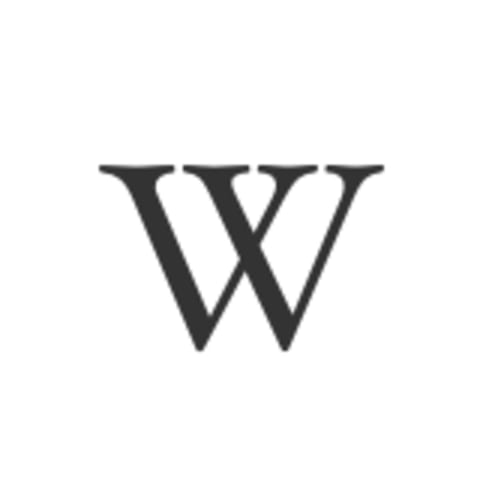
- Ver el informe original en su fuente
- Ver el informe en el Archivo de Internet
La DAO (Đ estilizada) era una organización autónoma digital descentralizada,[5] y una forma de fondo de capital de riesgo dirigido por inversores.[6]
La DAO tenía como objetivo proporcionar un nuevo modelo comercial descentralizado para org…
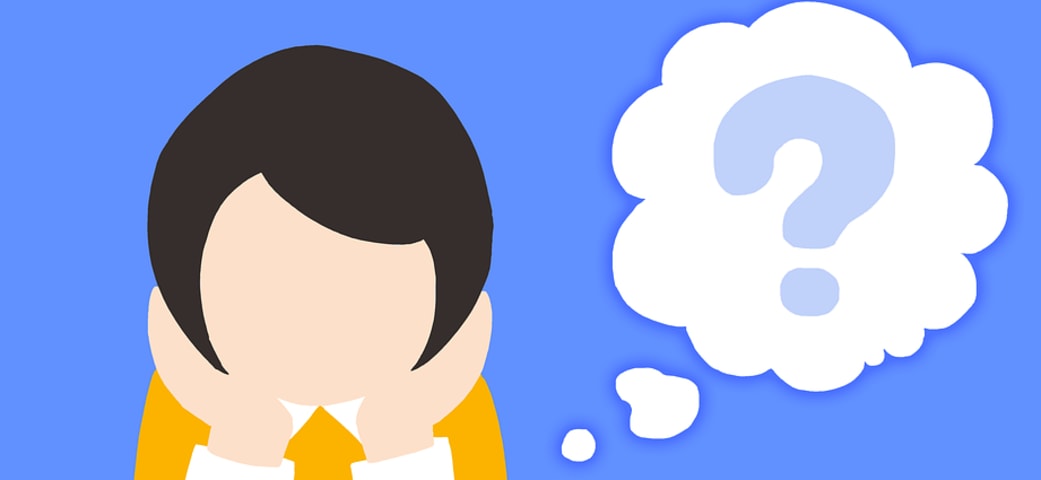
- Ver el informe original en su fuente
- Ver el informe en el Archivo de Internet
Hemos hecho todo lo posible para curar y aclarar los desarrollos de pirateo de DAO. Esta página se actualizará para reflejar nuevos desarrollos. No dude en señalar las incoherencias y sugerir actualizaciones.
[Lire cette FAQ en francés]
ACT…
Variantes
Incidentes Similares
Did our AI mess up? Flag the unrelated incidents
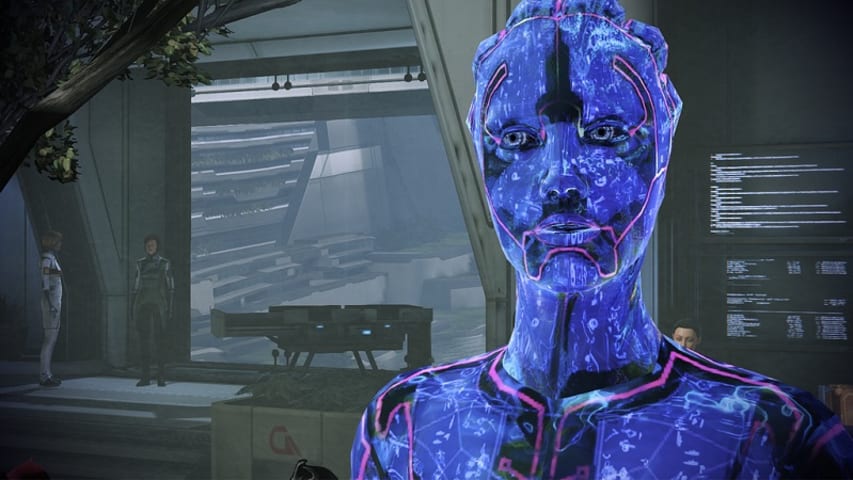
6 goof-ups that show AI is still in its diapers
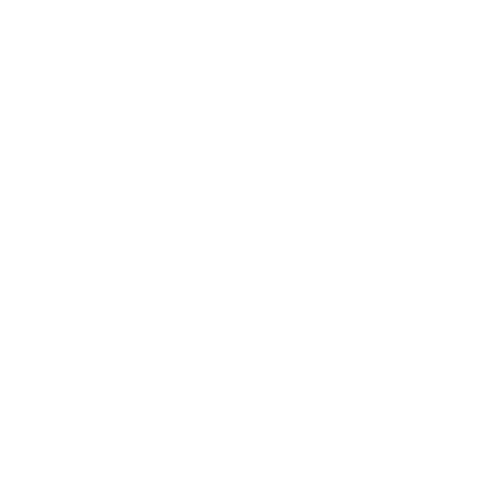
Danger, danger! 10 alarming examples of AI gone wild
Incidentes Similares
Did our AI mess up? Flag the unrelated incidents
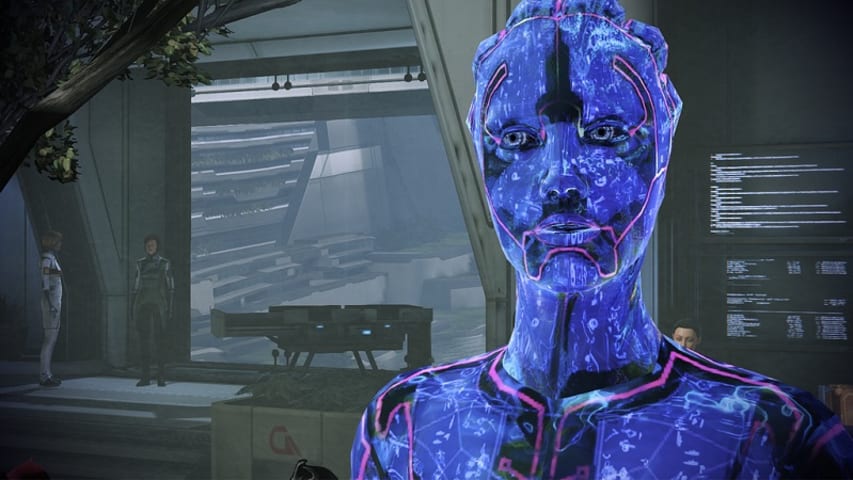
6 goof-ups that show AI is still in its diapers
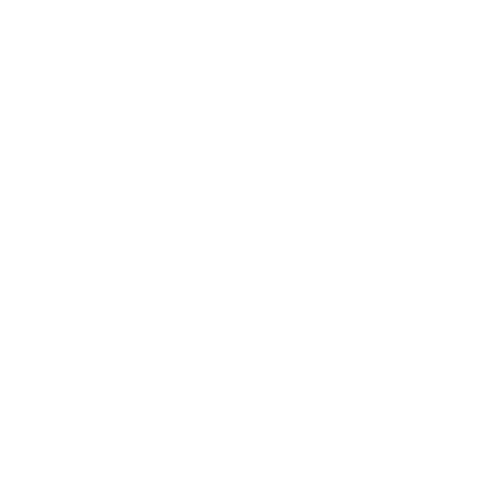