インシデント 74: translated-ja-Detroit Police Wrongfully Arrested Black Man Due To Faulty FRT
CSETv0 分類法のクラス
分類法の詳細Problem Nature
Specification, Assurance
Physical System
Software only
Level of Autonomy
High
Nature of End User
Amateur
Public Sector Deployment
Yes
Data Inputs
biometrics, images, camera footage
GMF 分類法のクラス
分類法の詳細Known AI Goal Snippets
(Snippet Text: On a Thursday afternoon in January, Robert Julian-Borchak Williams was in his office at an automotive supply company when he got a call from the Detroit Police Department telling him to come to the station to be arrested., Related Classifications: Face Recognition)
CSETv1 分類法のクラス
分類法の詳細Incident Number
74
Risk Subdomain
1.3. Unequal performance across groups
Risk Domain
- Discrimination and Toxicity
Entity
AI
Timing
Post-deployment
Intent
Unintentional
インシデントレポート
レポートタイムライン
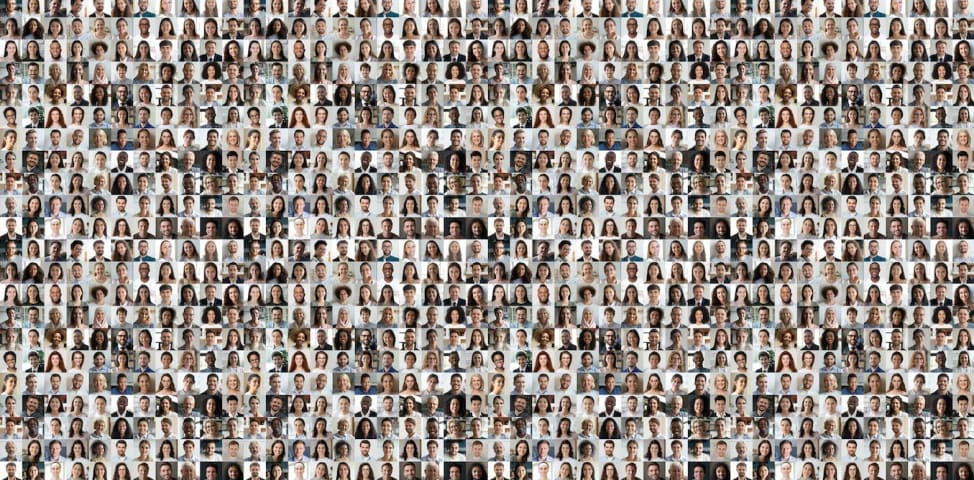
Detroit police wrongfully arrested Robert Julian-Borchak Williams in January 2020 for a shoplifting incident that had taken place two years earlier. Even though Williams had nothing to do with the incident, facial recognition technology use…
"Note: In response to this article, the Wayne County prosecutor’s office said that Robert Julian-Borchak Williams could have the case and his fingerprint data expunged. “We apologize,” the prosecutor, Kym L. Worthy, said in a statement, add…
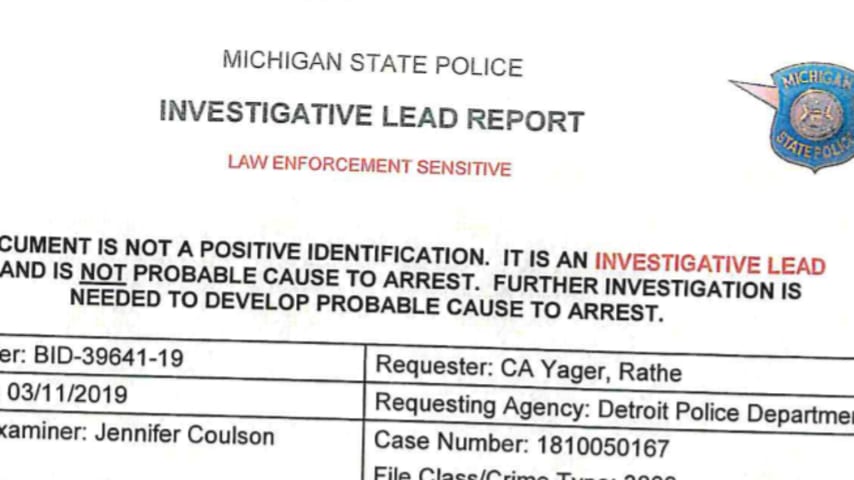
Updated 9:05 p.m. ET Wednesday
Police in Detroit were trying to figure out who stole five watches from a Shinola retail store. Authorities say the thief took off with an estimated $3,800 worth of merchandise.
Investigators pulled a security…
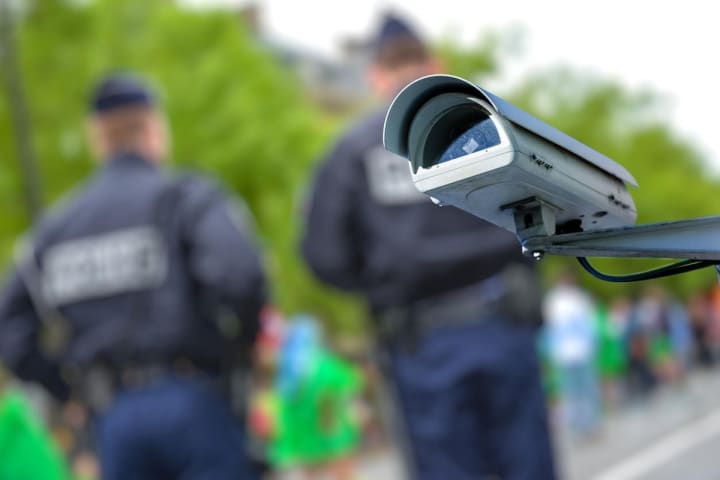
On Wednesday morning, the ACLU announced that it was filing a complaint against the Detroit Police Department on behalf of Robert Williams, a Black Michigan resident whom the group said is one of the first people falsely arrested due to fac…
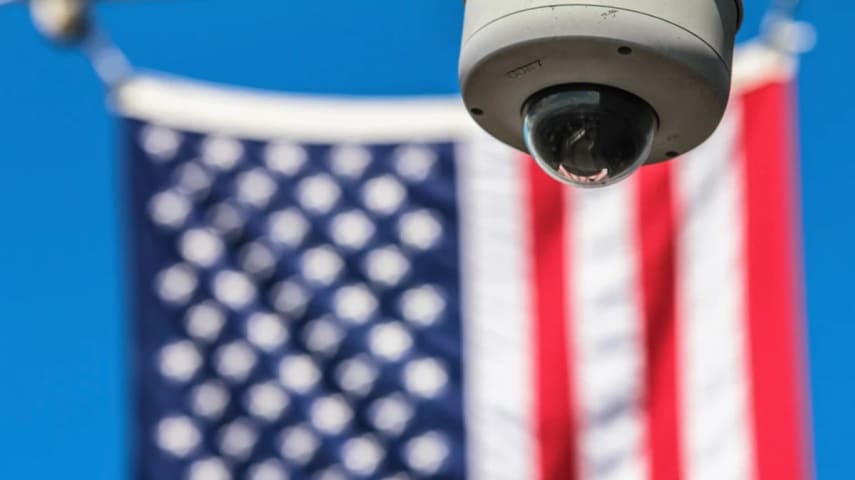
Detroit police have used highly unreliable facial recognition technology almost exclusively against Black people so far in 2020, according to the Detroit Police Department’s own statistics. The department’s use of the technology gained nati…
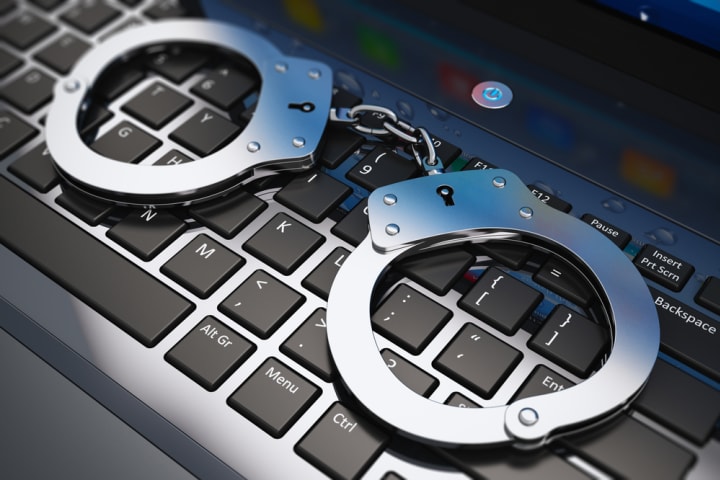
Racial bias and facial recognition. Black man in New Jersey arrested by police and spends ten days in jail after false face recognition match
Accuracy and racial bias concerns about facial recognition technology continue with the news of a …
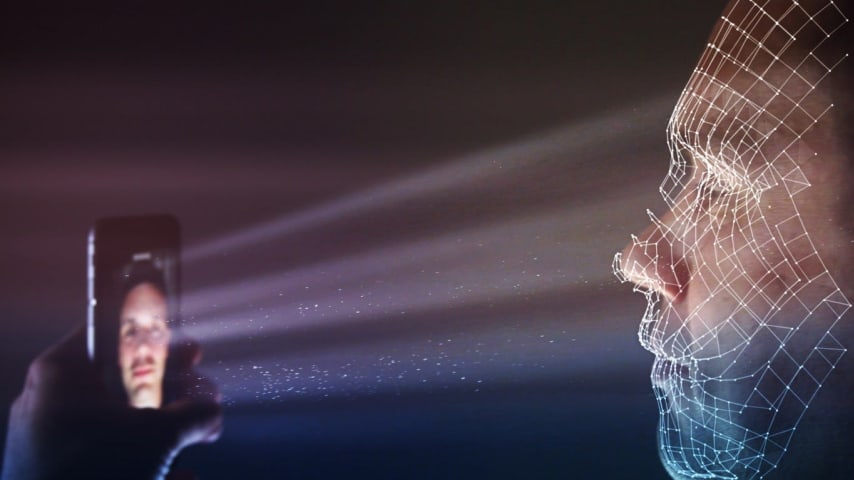
Teaneck just banned facial recognition technology for police. Here's why
Show Caption Hide Caption Facial recognition program that works even if you’re wearing a mask A Japanese company says they’ve developed a system that can bypass face c…
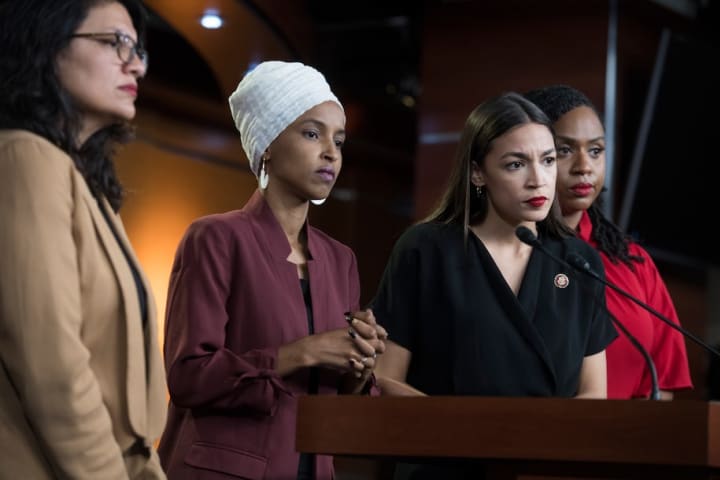
A Michigan man has sued Detroit police after he was wrongfully arrested and falsely identified as a shoplifting suspect by the department’s facial recognition software in one of the first lawsuits of its kind to call into question the contr…
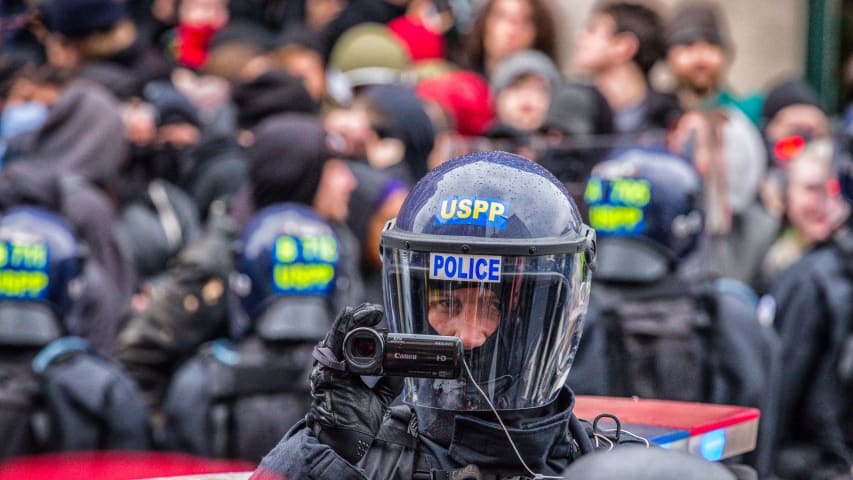
Since a Minneapolis police officer killed George Floyd in March 2020 and re-ignited massive Black Lives Matter protests, communities across the country have been re-thinking law enforcement, from granular scrutiny of the ways that police us…
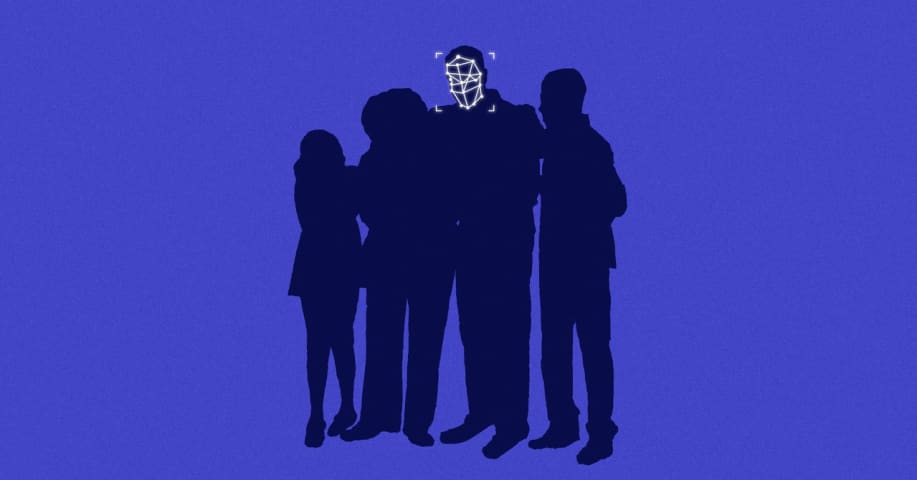
ROBERT WILLIAMS WAS doing yard work with his family one afternoon last August when his daughter Julia said they needed a family meeting immediately. Once everyone was inside the house, the 7-year-old girl closed all the blinds and curtains …
translated-ja-In January 2020, Robert Williams spent 30 hours in a Detroit jail because facial recognition technology suggested he was a criminal. The match was wrong, and Mr. Williams sued.
On Friday, as part of a legal settlement over his…
バリア ント
よく似たインシデント
Did our AI mess up? Flag the unrelated incidents
よく似たインシデント
Did our AI mess up? Flag the unrelated incidents