CSETv1 分類法のクラス
分類法の詳細Incident Number
7
AI Tangible Harm Level Notes
It is unclear if any of the Wikipedia bots under study relies on machine learning technology, but it is unlikely. Nobody experienced any harm.
Special Interest Intangible Harm
no
Date of Incident Year
2001
CSETv0 分類法のクラス
分類法の詳細Problem Nature
Specification, Robustness, Assurance
Physical System
Software only
Level of Autonomy
High
Nature of End User
Amateur
Public Sector Deployment
No
Data Inputs
Wikipedia articles, edits from other bots
Risk Subdomain
7.3. Lack of capability or robustness
Risk Domain
- AI system safety, failures, and limitations
Entity
AI
Timing
Post-deployment
Intent
Unintentional
インシデントレポート
レポートタイムライン
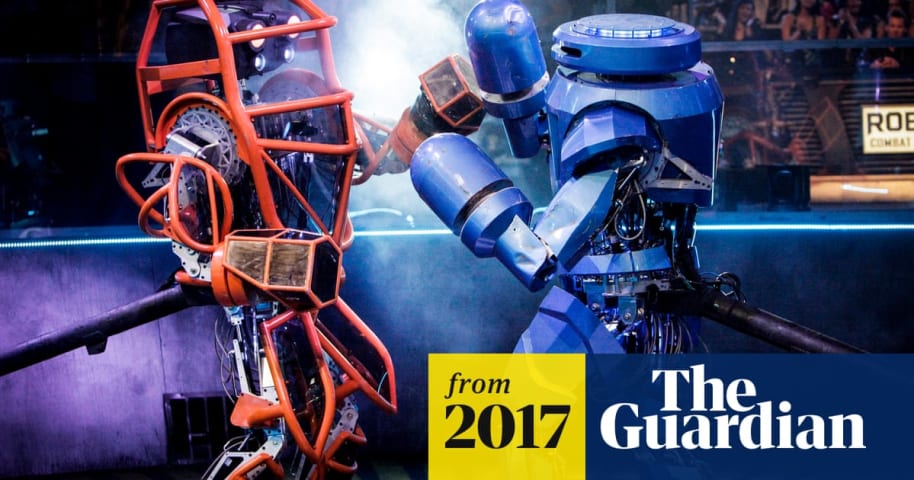
For many it is no more than the first port of call when a niggling question raises its head. Found on its pages are answers to mysteries from the fate of male anglerfish, the joys of dorodango, and the improbable death of Aeschylus.
But ben…
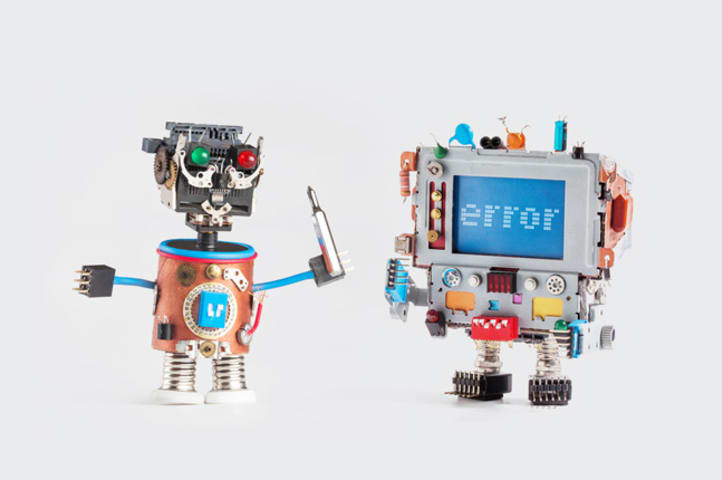
Analysis An investigation into Wikipedia bots has confirmed the automated editing software can be just as pedantic and petty as humans are – often engaging in online spats that can continue for years.
What's interesting is that bots behave …
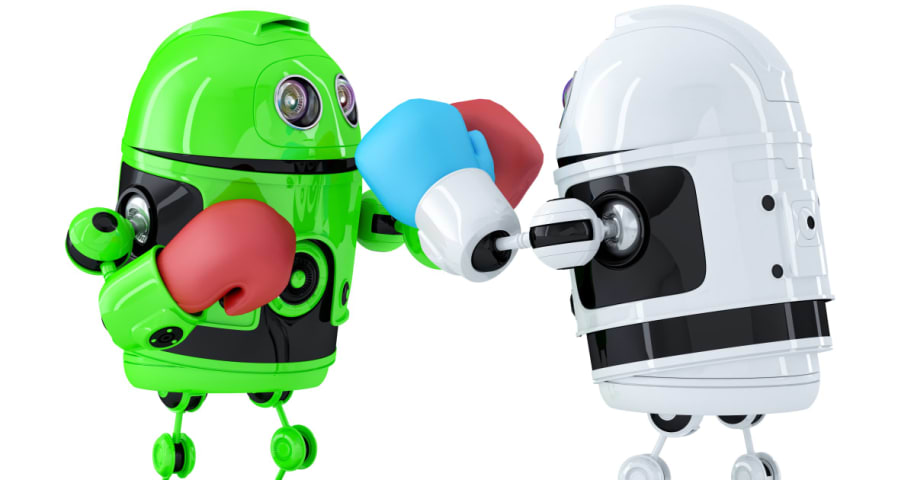
It turns out Wikipedia's automated edit 'bots' have been waging a cyber-war between each other for over a decade by changing each other's corrections -- and it's getting worse.
Researchers at the University of Oxford in the United Kingdom r…

Wiki Bots That Feud for Years Highlight the Troubled Future of AI
The behavior of bots is often unpredictable and sometimes leads them to produce errors over and over again in a potentially infinite feedback loop.
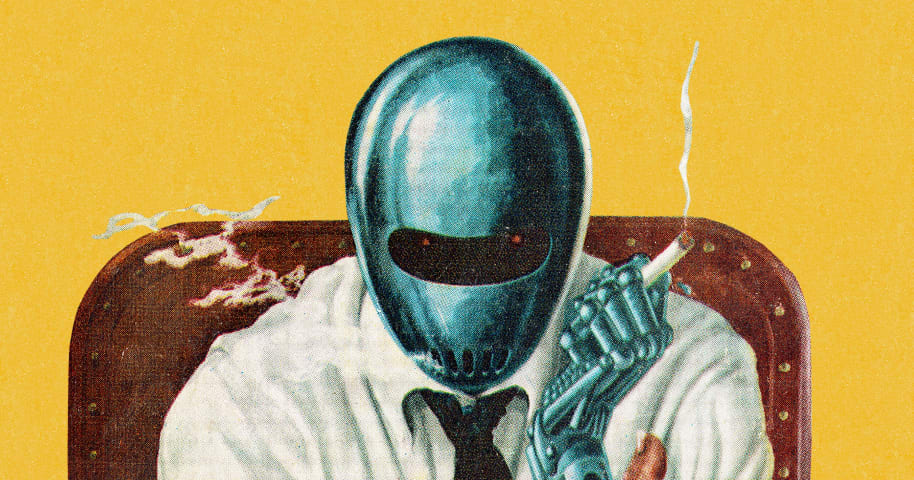
Getty Images
No one saw the crisis coming: a coordinated vandalistic effort to insert Squidward references into articles totally unrelated to Squidward. In 2006, Wikipedia was really starting to get going, and really couldn’t afford to have…
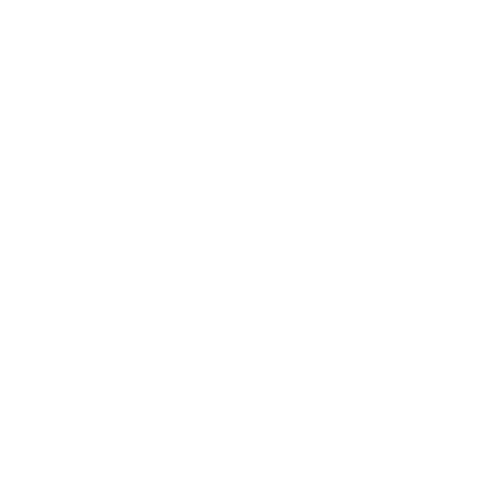
Science fiction is lousy with tales of artificial intelligence run amok. There's HAL 9000, of course, and the nefarious Skynet system from the "Terminator" films. Last year, the sinister AI Ultron came this close to defeating the Avengers, …
バリアント
よく似たインシデント
Did our AI mess up? Flag the unrelated incidents
よく似たインシデント
Did our AI mess up? Flag the unrelated incidents