CSETv0 分類法のクラス
分類法の詳細Public Sector Deployment
No
Infrastructure Sectors
Healthcare and public health
Lives Lost
No
Intent
Unclear
Near Miss
Unclear/unknown
Ending Date
1996-04-03
CSETv1 分類法のクラス
分類法の詳細Incident Number
42
Estimated Date
No
Lives Lost
0
Injuries
0
Estimated Harm Quantities
No
There is a potentially identifiable specific entity that experienced the harm
No
Risk Subdomain
5.2. Loss of human agency and autonomy
Risk Domain
- Human-Computer Interaction
Entity
Human
Timing
Pre-deployment
Intent
Intentional
インシデントレポート
レポートタイムライン
The National Residency Matching Program as a Labor Market
[Pulse: Communication]
Roth, Alvin E. PhD
Mellon Professor of Economics, Department of Economics, University of Pittsburgh.
Graphics Table 1
Although medical students are unaccustome…
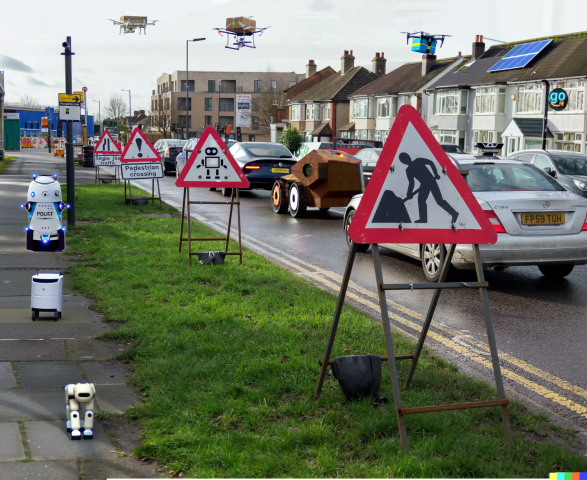
The following former incidents have been converted to "issues" following an update to the incident definition and ingestion criteria.
21: Tougher Turing Test Exposes Chatbots’ Stupidity
Description: The 2016 Winograd Schema Challenge highli…
バリアント
よく似たインシデント
Did our AI mess up? Flag the unrelated incidents
よく似たインシデント
Did our AI mess up? Flag the unrelated incidents