Incidente 65: translated-es-Reinforcement Learning Reward Functions in Video Games
Entidades
Ver todas las entidadesClasificaciones de la Taxonomía CSETv0
Detalles de la TaxonomíaProblem Nature
Specification
Physical System
Software only
Level of Autonomy
Unclear/unknown
Nature of End User
Expert
Public Sector Deployment
No
Data Inputs
Universe software training
Clasificaciones de la Taxonomía CSETv1
Detalles de la TaxonomíaIncident Number
65
Special Interest Intangible Harm
no
Date of Incident Year
2016
Date of Incident Month
12
Date of Incident Day
21
Estimated Date
Yes
Risk Subdomain
7.1. AI pursuing its own goals in conflict with human goals or values
Risk Domain
- AI system safety, failures, and limitations
Entity
AI
Timing
Post-deployment
Intent
Unintentional
Informes del Incidente
Cronología de Informes
En OpenAI, recientemente comenzamos a usar Universe, nuestro software para medir y entrenar agentes de IA, para realizar nuevos experimentos de RL. A veces, estos experimentos ilustran algunos de los problemas con RL tal como se practica ac…
Variantes
Incidentes Similares
Did our AI mess up? Flag the unrelated incidents
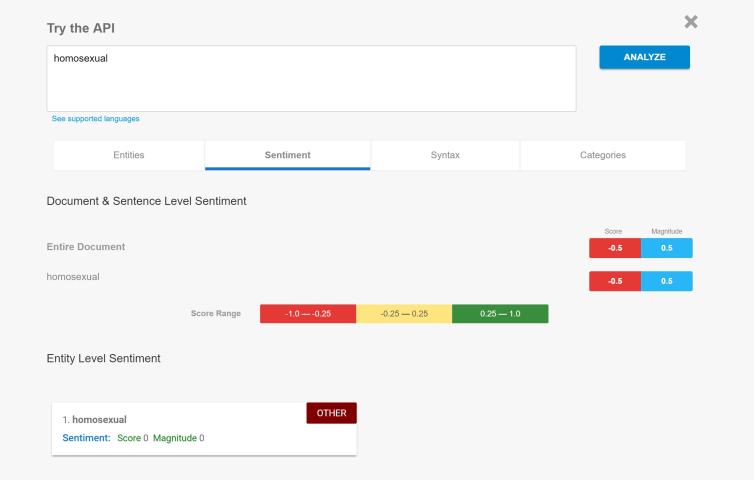
Biased Sentiment Analysis
Gender Biases in Google Translate
Incidentes Similares
Did our AI mess up? Flag the unrelated incidents
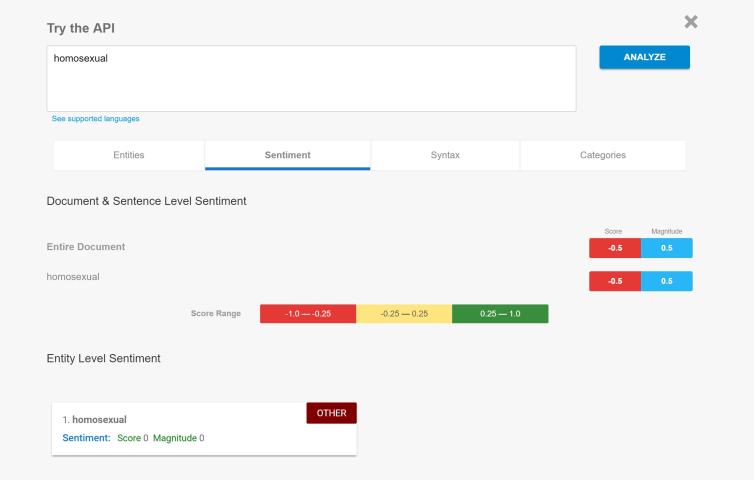