Description: A study by the University of Toronto, the Vector Institute, and MIT showed the input databases that trained AI systems used to classify chest X-rays led the systems to show gender, socioeconomic, and racial biases.
Entities
View all entitiesAlleged: Google , Qure.ai , Aidoc and DarwinAI developed an AI system deployed by Mount Sinai Hospitals, which harmed patients of minority groups , low-income patients , female patients , Hispanic patients and patients with Medicaid insurance.
CSETv1 Taxonomy Classifications
Taxonomy DetailsIncident Number
The number of the incident in the AI Incident Database.
81
CSETv0 Taxonomy Classifications
Taxonomy DetailsProblem Nature
Indicates which, if any, of the following types of AI failure describe the incident: "Specification," i.e. the system's behavior did not align with the true intentions of its designer, operator, etc; "Robustness," i.e. the system operated unsafely because of features or changes in its environment, or in the inputs the system received; "Assurance," i.e. the system could not be adequately monitored or controlled during operation.
Specification
Physical System
Where relevant, indicates whether the AI system(s) was embedded into or tightly associated with specific types of hardware.
Software only
Level of Autonomy
The degree to which the AI system(s) functions independently from human intervention. "High" means there is no human involved in the system action execution; "Medium" means the system generates a decision and a human oversees the resulting action; "low" means the system generates decision-support output and a human makes a decision and executes an action.
Unclear/unknown
Nature of End User
"Expert" if users with special training or technical expertise were the ones meant to benefit from the AI system(s)’ operation; "Amateur" if the AI systems were primarily meant to benefit the general public or untrained users.
Expert
Public Sector Deployment
"Yes" if the AI system(s) involved in the accident were being used by the public sector or for the administration of public goods (for example, public transportation). "No" if the system(s) were being used in the private sector or for commercial purposes (for example, a ride-sharing company), on the other.
No
Data Inputs
A brief description of the data that the AI system(s) used or were trained on.
medical imagery databases
Risk Subdomain
A further 23 subdomains create an accessible and understandable classification of hazards and harms associated with AI
1.3. Unequal performance across groups
Risk Domain
The Domain Taxonomy of AI Risks classifies risks into seven AI risk domains: (1) Discrimination & toxicity, (2) Privacy & security, (3) Misinformation, (4) Malicious actors & misuse, (5) Human-computer interaction, (6) Socioeconomic & environmental harms, and (7) AI system safety, failures & limitations.
- Discrimination and Toxicity
Entity
Which, if any, entity is presented as the main cause of the risk
Human
Timing
The stage in the AI lifecycle at which the risk is presented as occurring
Pre-deployment
Intent
Whether the risk is presented as occurring as an expected or unexpected outcome from pursuing a goal
Unintentional
Incident Reports
Reports Timeline
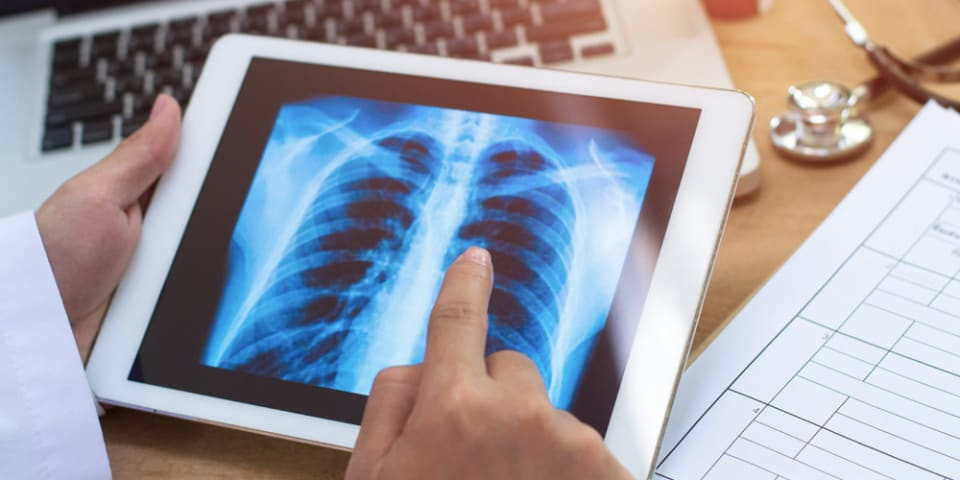
Google and startups like Qure.ai, Aidoc, and DarwinAI are developing AI and machine learning systems that classify chest X-rays to help identify conditions like fractures and collapsed lungs. Several hospitals, including Mount Sinai, have p…
Variants
A "variant" is an incident that shares the same causative factors, produces similar harms, and involves the same intelligent systems as a known AI incident. Rather than index variants as entirely separate incidents, we list variations of incidents under the first similar incident submitted to the database. Unlike other submission types to the incident database, variants are not required to have reporting in evidence external to the Incident Database. Learn more from the research paper.
Similar Incidents
Did our AI mess up? Flag the unrelated incidents
Similar Incidents
Did our AI mess up? Flag the unrelated incidents