Description: Heriot-Watt Univeristy in Scotland developed an artificially intelligent grocery store robot, Fabio, who provided unhelpful answers to customer's questions and "scared away" multiple customers, according to the grocery store Margiotta.
Entities
View all entitiesAlleged: Heriot-Watt University developed an AI system deployed by Heriot-Watt University and Margiotta, which harmed Store Patrons.
CSETv0 Taxonomy Classifications
Taxonomy DetailsProblem Nature
Indicates which, if any, of the following types of AI failure describe the incident: "Specification," i.e. the system's behavior did not align with the true intentions of its designer, operator, etc; "Robustness," i.e. the system operated unsafely because of features or changes in its environment, or in the inputs the system received; "Assurance," i.e. the system could not be adequately monitored or controlled during operation.
Specification, Assurance
Physical System
Where relevant, indicates whether the AI system(s) was embedded into or tightly associated with specific types of hardware.
Vehicle/mobile robot, Software only
Level of Autonomy
The degree to which the AI system(s) functions independently from human intervention. "High" means there is no human involved in the system action execution; "Medium" means the system generates a decision and a human oversees the resulting action; "low" means the system generates decision-support output and a human makes a decision and executes an action.
Medium
Nature of End User
"Expert" if users with special training or technical expertise were the ones meant to benefit from the AI system(s)’ operation; "Amateur" if the AI systems were primarily meant to benefit the general public or untrained users.
Expert
Public Sector Deployment
"Yes" if the AI system(s) involved in the accident were being used by the public sector or for the administration of public goods (for example, public transportation). "No" if the system(s) were being used in the private sector or for commercial purposes (for example, a ride-sharing company), on the other.
No
Data Inputs
A brief description of the data that the AI system(s) used or were trained on.
Customer requests
CSETv1 Taxonomy Classifications
Taxonomy DetailsIncident Number
The number of the incident in the AI Incident Database.
64
Special Interest Intangible Harm
An assessment of whether a special interest intangible harm occurred. This assessment does not consider the context of the intangible harm, if an AI was involved, or if there is characterizable class or subgroup of harmed entities. It is also not assessing if an intangible harm occurred. It is only asking if a special interest intangible harm occurred.
no
Date of Incident Year
The year in which the incident occurred. If there are multiple harms or occurrences of the incident, list the earliest. If a precise date is unavailable, but the available sources provide a basis for estimating the year, estimate. Otherwise, leave blank.
Enter in the format of YYYY
2018
Estimated Date
“Yes” if the data was estimated. “No” otherwise.
Yes
Multiple AI Interaction
“Yes” if two or more independently operating AI systems were involved. “No” otherwise.
no
Embedded
“Yes” if the AI is embedded in a physical system. “No” if it is not. “Maybe” if it is unclear.
yes
Incident Reports
Reports Timeline
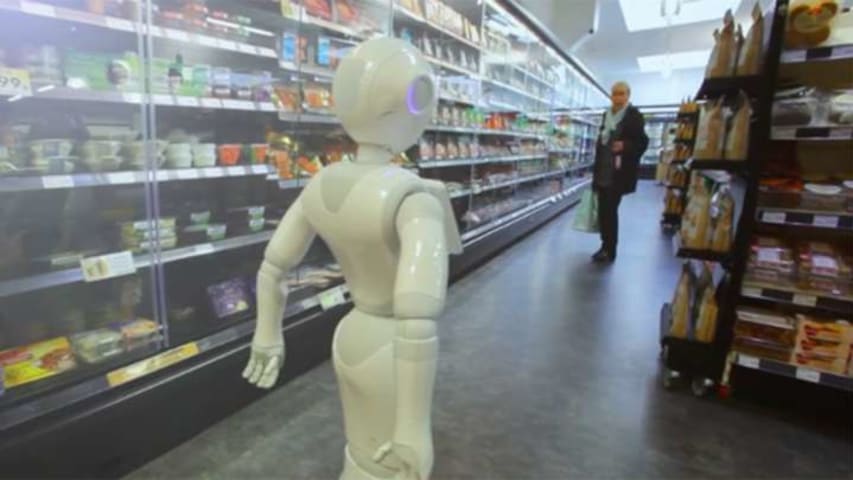
iflscience.com · 2018
- View the original report at its source
- View the report at the Internet Archive
Every few months there's a story warning us that robots will take over our jobs within five, 10, or 20 years. You don't get a lot of stories about robots taking over jobs right here and now. So what would happen if robots were hired now? Ar…
Variants
A "variant" is an incident that shares the same causative factors, produces similar harms, and involves the same intelligent systems as a known AI incident. Rather than index variants as entirely separate incidents, we list variations of incidents under the first similar incident submitted to the database. Unlike other submission types to the incident database, variants are not required to have reporting in evidence external to the Incident Database. Learn more from the research paper.
Similar Incidents
Did our AI mess up? Flag the unrelated incidents
Similar Incidents
Did our AI mess up? Flag the unrelated incidents