Entities
View all entitiesCSETv1 Taxonomy Classifications
Taxonomy DetailsIncident Number
461
Special Interest Intangible Harm
yes
Date of Incident Year
2023
Date of Incident Month
01
Date of Incident Day
Estimated Date
No
Risk Subdomain
1.1. Unfair discrimination and misrepresentation
Risk Domain
- Discrimination and Toxicity
Entity
AI
Timing
Post-deployment
Intent
Unintentional
Incident Reports
Reports Timeline
Government agencies around the world use data-driven algorithms to allocate enforcement resources. Even when such algorithms are formally neutral with respect to protected characteristics like race, there is widespread concern that they can…
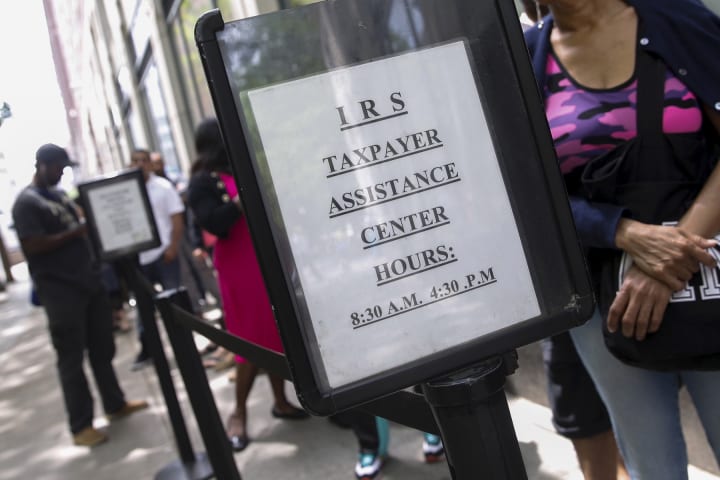
Researchers have long wondered if the IRS uses its audit powers equitably. And now we have learned that it does not.
Black taxpayers receive IRS audit notices at least 2.9 times (and perhaps as much as 4.7 times) more often than non-Black t…
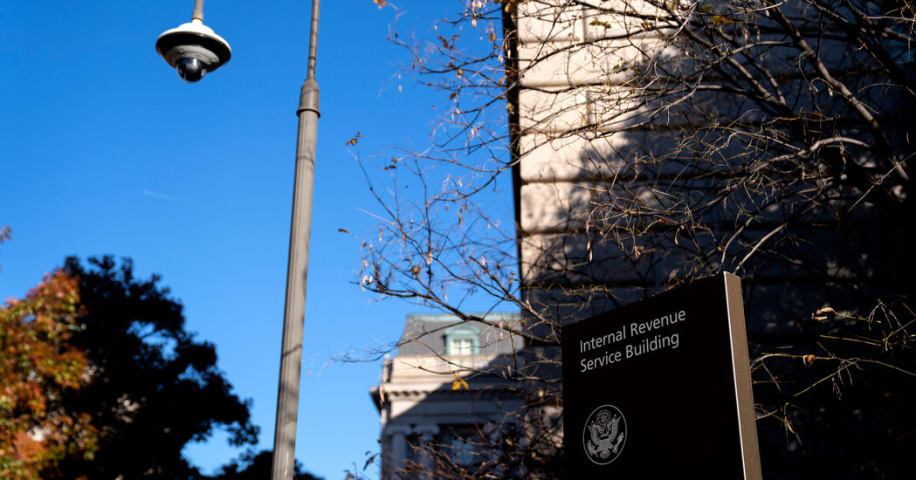
WASHINGTON — Black taxpayers are at least three times as likely to be audited by the Internal Revenue Service as other taxpayers, even after accounting for the differences in the types of returns each group is most likely to file, a team of…
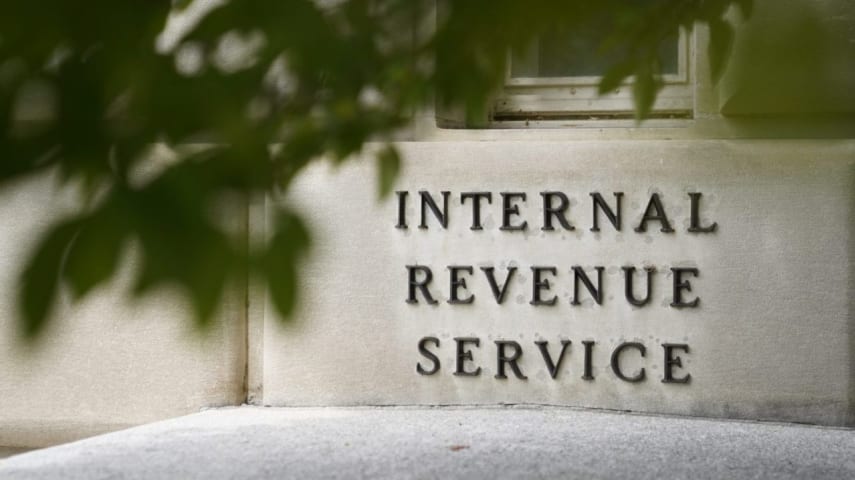
A new report published Monday found that the IRS audits Black taxpayers at a significantly higher rate than non-Black taxpayers.
The paper, published by Stanford’s Institute for Economic Policy Research, said that despite the IRS’s “race-bl…
Variants
Similar Incidents
Did our AI mess up? Flag the unrelated incidents
Similar Incidents
Did our AI mess up? Flag the unrelated incidents