Description: Emotion detection tools by Face++ and Microsoft's Face API allegedly scored smiling or defaulted ambiguous facial photos for Black faces as negative emotion more often than for white faces.
Entities
View all entitiesIncident Stats
CSETv1 Taxonomy Classifications
Taxonomy DetailsIncident Number
The number of the incident in the AI Incident Database.
454
Special Interest Intangible Harm
An assessment of whether a special interest intangible harm occurred. This assessment does not consider the context of the intangible harm, if an AI was involved, or if there is characterizable class or subgroup of harmed entities. It is also not assessing if an intangible harm occurred. It is only asking if a special interest intangible harm occurred.
yes
Date of Incident Year
The year in which the incident occurred. If there are multiple harms or occurrences of the incident, list the earliest. If a precise date is unavailable, but the available sources provide a basis for estimating the year, estimate. Otherwise, leave blank.
Enter in the format of YYYY
2018
Date of Incident Month
The month in which the incident occurred. If there are multiple harms or occurrences of the incident, list the earliest. If a precise date is unavailable, but the available sources provide a basis for estimating the month, estimate. Otherwise, leave blank.
Enter in the format of MM
12
Date of Incident Day
The day on which the incident occurred. If a precise date is unavailable, leave blank.
Enter in the format of DD
Estimated Date
“Yes” if the data was estimated. “No” otherwise.
No
Incident Reports
Reports Timeline
papers.ssrn.com · 2018
- View the original report at its source
- View the report at the Internet Archive
Abstract
The practical applications of artificial intelligence are expanding into various elements of society, leading to a growing interest in the potential biases of such algorithms. Facial analysis, one application of artificial intellig…
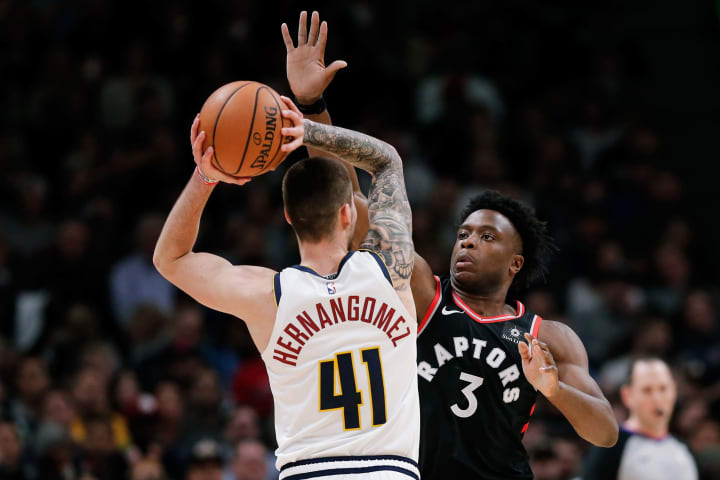
theconversation.com · 2019
- View the original report at its source
- View the report at the Internet Archive
Facial recognition technology has progressed to point where it now interprets emotions in facial expressions. This type of analysis is increasingly used in daily life. For example, companies can use facial recognition software to help with …
Variants
A "variant" is an incident that shares the same causative factors, produces similar harms, and involves the same intelligent systems as a known AI incident. Rather than index variants as entirely separate incidents, we list variations of incidents under the first similar incident submitted to the database. Unlike other submission types to the incident database, variants are not required to have reporting in evidence external to the Incident Database. Learn more from the research paper.