Description: Pepsi's number generation system determining daily winners in its Number Fever promotion in the Philippines mistakenly produced a number held by thousands which resulted in riots, deaths, conspiracy theories, and decades of lawsuits.
Entities
View all entitiesIncident Stats
Risk Subdomain
A further 23 subdomains create an accessible and understandable classification of hazards and harms associated with AI
7.3. Lack of capability or robustness
Risk Domain
The Domain Taxonomy of AI Risks classifies risks into seven AI risk domains: (1) Discrimination & toxicity, (2) Privacy & security, (3) Misinformation, (4) Malicious actors & misuse, (5) Human-computer interaction, (6) Socioeconomic & environmental harms, and (7) AI system safety, failures & limitations.
- AI system safety, failures, and limitations
Entity
Which, if any, entity is presented as the main cause of the risk
Human
Timing
The stage in the AI lifecycle at which the risk is presented as occurring
Post-deployment
Intent
Whether the risk is presented as occurring as an expected or unexpected outcome from pursuing a goal
Unintentional
Incident Reports
Reports Timeline
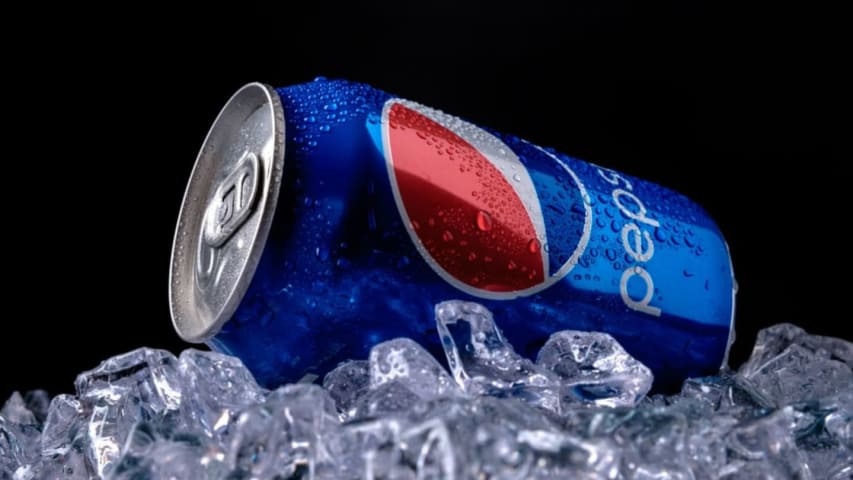
On May 25, 1992, the Channel 2 News program in Manila, Philippines aired a segment that had been running since February of that year. Each night, the station alerted viewers to the day’s winning number in Pepsi’s Number Fever promotion. Buy…
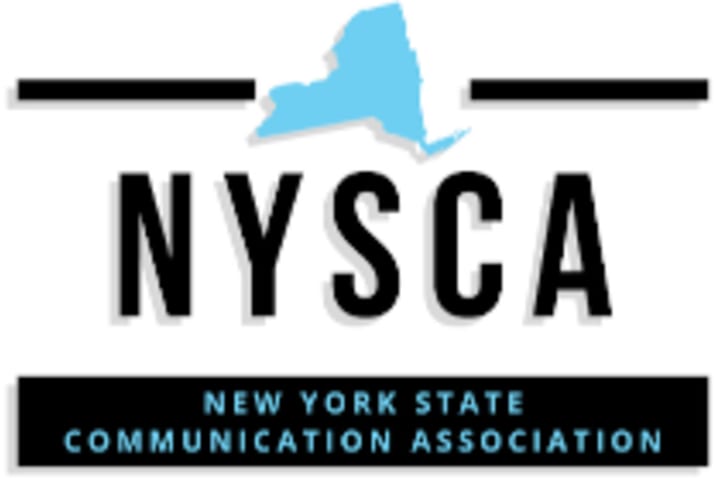
In 1992, Pepsi created a marketing ploy to increase interest in its products in the Philippines. The game was aptly called “Number Fever,” and participants had to look at the number printed underneath the cap of their soft drink bottle and …
Variants
A "variant" is an incident that shares the same causative factors, produces similar harms, and involves the same intelligent systems as a known AI incident. Rather than index variants as entirely separate incidents, we list variations of incidents under the first similar incident submitted to the database. Unlike other submission types to the incident database, variants are not required to have reporting in evidence external to the Incident Database. Learn more from the research paper.
Similar Incidents
Did our AI mess up? Flag the unrelated incidents
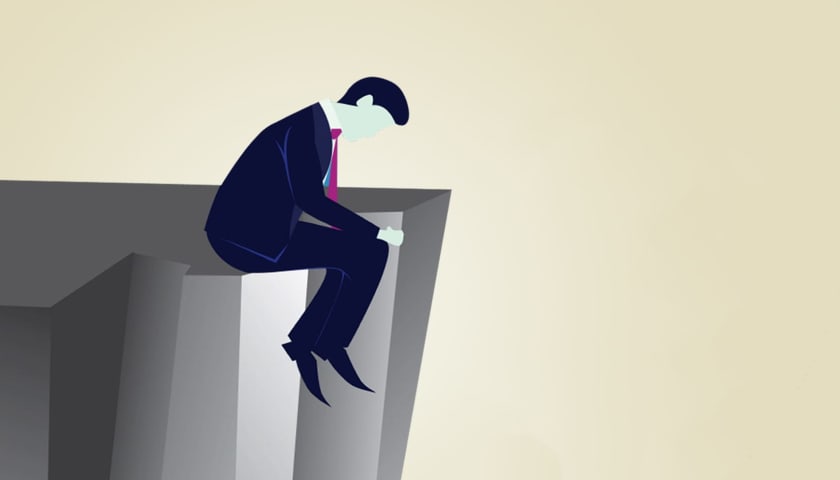
Amazon Alexa Responding to Environmental Inputs
· 35 reports
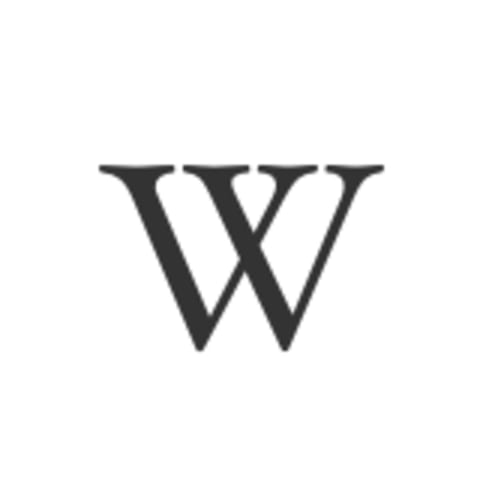
Nuclear False Alarm
· 27 reports
Similar Incidents
Did our AI mess up? Flag the unrelated incidents
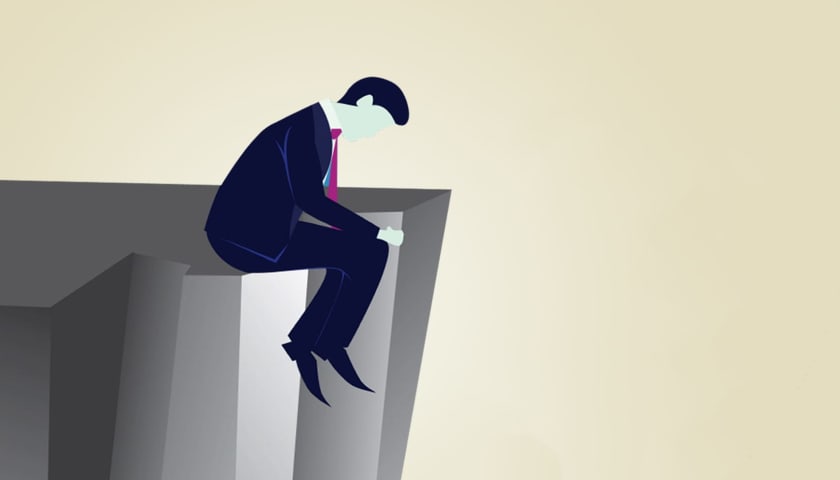
Amazon Alexa Responding to Environmental Inputs
· 35 reports
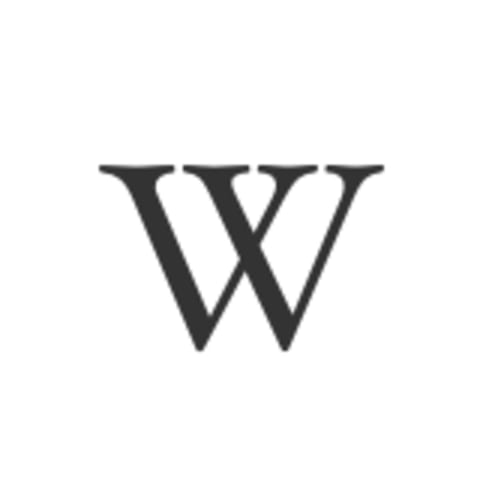
Nuclear False Alarm
· 27 reports